PENENTUAN PARAMETER LEARNING RATE SELAMA PEMBELAJARAN JARINGAN SYARAF TIRUAN BACKPROPAGATION MENGGUNAKAN ALGORITMA GENETIKA
DOI:
https://doi.org/10.47111/jti.v14i2.1141Keywords:
Artificial Neural Network Genetic Algorithm Backpropagation Local minima SSEAbstract
One of the weakness in backpropagation Artificial neural network(ANN) is being stuck in local minima. Learning rate parameter is an important parameter in order to determine how fast the ANN Learning. This research is conducted to determine a method of finding the value of learning rate parameter using a genetic algorithm when neural network learning stops and the error value is not reached the stopping criteria or has not reached the convergence. Genetic algorithm is used to determine the value of learning rate used is based on the calculation of the fitness function with the input of the ANN weights, gradient error, and bias. The calculation of the fitness function will produce an error value of each learning rate which represents each candidate solutions or individual genetic algorithms. Each individual is determined by sum of squared error value. One with the smallest SSE is the best individual. The value of learning rate has chosen will be used to continue learning so that it can lower the value of the error or speed up the learning towards convergence.
The final result of this study is to provide a new solution to resolve the problem in the backpropagation learning that often have problems in determining the learning parameters. These results indicate that the method of genetic algorithms can provide a solution for backpropagation learning in order to decrease the value of SSE when learning of ANN has been static in large error conditions, or stuck in local minima
Downloads
References
Rojas, R., 1996, Neural Networks: A Systematic Introduction, New York, Springer.
Suhendra, C. D., & Wardoyo, R. (2015). Penentuan Arsitektur Jaringan Syaraf Tiruan Backpropagation (Bobot Awal dan Bias Awal) Menggunakan Algoritma Genetika. IJCCS (Indonesian Journal of Computing and Cybernetics Systems), 9(1), 77.
Nawi,N,M., Khan, A., Rehman, M, Z., 2013, A New Backpropagation Neural Network Optimized with Cuckoo Search Algorithm, Computational Science and Its Applications-ICCSA, volume 7971, pp 413-426.
Chang, Y., Lin, J., Sieh, J., Abbod, M,F., 2012, Optimization the Initial Weights of Artificial Neural Networks via Genetic Algorithm Applied to Hip Bone Fracture Prediction, Taiwan, Hindawi Publishing Corporation Advances in Fuzzy Systems, 951247, 2012, 9.
Nuzly, H., 2006 Particel Swarm Optimization For Neural Network Learning Enhancment, Tesis, Faculty of Computer Science and Information System Univesiti Teknologi Malaysia.
Yamamoto, K., Koakutsu, S., Okamoto, T. and Hirata, H. (2011), Fast backpropagation learning using optimization of learning rate for pulsed neural networks. Electron. Comm. Jpn., 94: 27-34.
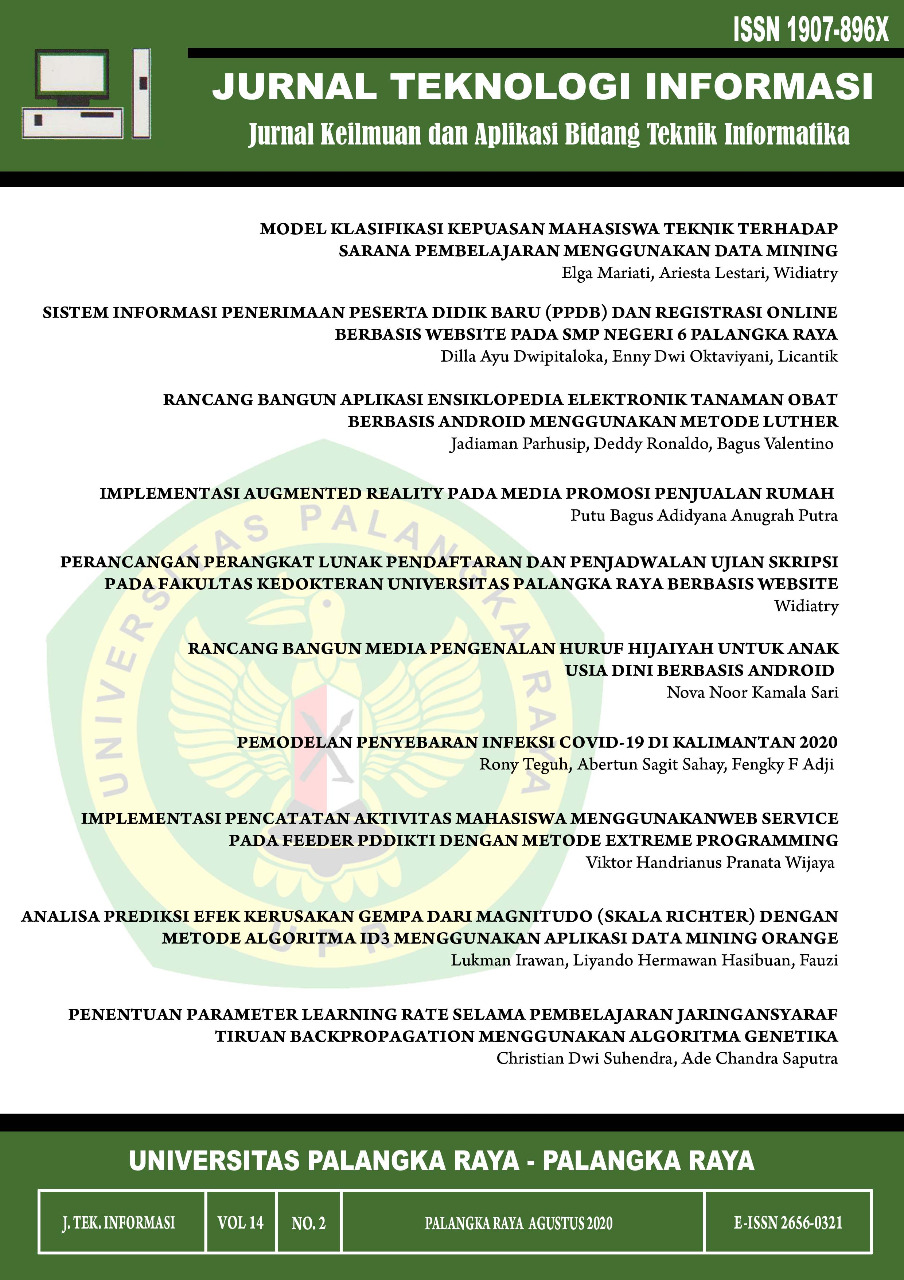